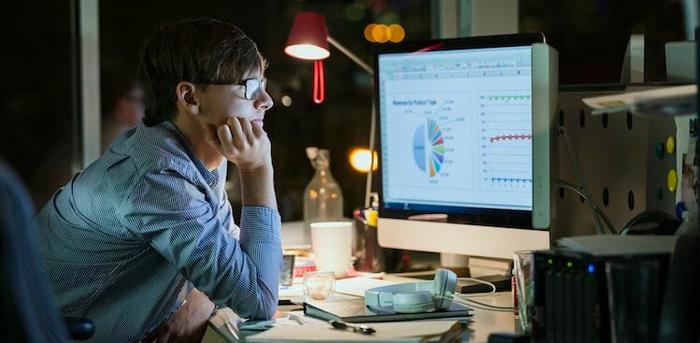
In the climax of the Academy Award-nominated film Hidden Figures, mathematician Katherine Johnson is called to verify calculations for the landing coordinates of John Glenn’s space capsule, Friendship 7. Technology has just replaced human computers, the data-calculating people who completed complex equations before the computer system’s advent, but the data from the machine had discrepancies that needed to be solved by a person.
That was data science in 1961. These days, things are a little different. Complex data collection systems enable companies in every sector to learn more about their businesses, clients, and future prospects. But much like in Hidden Figures, people are still needed to find important truths from within the data.
Here's the scoop on how we use data science every day and the essential skills you need to be successful as a data scientist, engineer, or analyst.
Data Science Is Everywhere
The potential for data scientists far beyond finance and tech industries is flourishing. “There’s a growing realization across all sectors that data science skills have become essential for competing and improving in today’s marketplace,” says Michael Galvin, executive director of Data Science Corporate Training for Metis, a data science skills training company that works with individuals and businesses.
Think about cookies. No, not the ones you dip in milk—the powerful data gathering tools that help data analysts, scientists, and engineers learn about consumer web habits and inform the algorithms around those “how’d-they-know-I-was just-thinking-of-that?!” ads we’re shown on Facebook. Their goal? To assess consumer interests and behavior and use those analytics to help make key business decisions—for companies across all sectors.
“There’s a broader awareness of data science in the mainstream. Affecting everything from Amazon purchases to Netflix binges, data science is touching more people than ever before,” says Galvin.
How You Fit In
With the growth in data science fields, there’s been an increased overlap between the roles of data scientist, data analyst, and modeler.
But according to Dr. Flavio Villanustre, Vice President of Technology and HPCC Systems for LexisNexis Risk Solutions, the distinction between various positions is actually quite unique—and presents opportunities for those gifted in specific areas.
“Data analysts traditionally specialize in data manipulation techniques, which require training in everything from query languages to graphical data models,” says Villanustre. “Meanwhile modelers analyze numerical data for correlations and patterns.”
When it comes to data science, Villanustre explains that ideal candidates should showcase a superset of these two types of skills compounded with domain and business knowledge. “Data scientists usually possess deeper knowledge than the data analyst about programming techniques and broader knowledge than statistical modelers about data analytical methodologies using more sophisticated techniques.”
When applying to these positions, it’s important to note what tasks a company is really looking to have performed.
“The buzz around data science has resulted in many companies hiring data scientists to do a data analyst’s job, who ends up cleansing and preparing data and spending very little time doing actual data science,” explains Nick Kramer, Senior Director of Data and Analytics at SSA & Company, a management consulting firm that specializes in transforming big data analytics into operations for companies.
New tools are allowing analytics models to be created by those with lower expertise levels, so diversified, related skills like business knowledge and effective communication skills are important to set job seekers apart. When interviewing, be sure to ask questions to hone in on exactly what a company is seeking—then showcase your strengths accordingly.
What You Need to Be Successful
The old adage about not seeing the forest for the trees is an important thing to remember when working as a data scientist, analyst, or engineer. While the accuracy of core data is important, so is acknowledging the overarching picture of the problems a company hopes to solve.
“There’s a tendency among data scientists to overcomplicate things and get sucked into a black hole of details,” warns Galvin. “Instead, they should think about the business problem they’re trying to solve, get something working, and then iterate.”
Moreover, an interest in what you’re doing—as is true of any job—is essential as well.
“Companies work with different kinds of data (like images, text, and financial data) on different problems. You need to be interested in and understand the kind of data you’ll be working with in order to succeed,” said Galvin. “For example, data scientists who work with medical images aren’t typically doctors themselves, but their end user or client will be a doctor. Can you understand what problems they’re trying to solve? Are you interested in solving those problems?”
And then there’s communication. It’s said that data scientists, analysts, and engineers speak their own language, but to be successful in a workplace, you need to be able to communicate clearly with those who will utilize and benefit most from your skills.
“Collaborating with business stakeholders is increasingly important,” said Kramer.
Data science and its related careers have come a long way from the 1960s when NASA needed human computers to harness and verify the work of new computer machines. But brilliant minds interested in how data can shape how we live, work, and do business are still as essential as ever—without a human expert to interpret both the inputs and the results, data science could be wildly misused, or just plain confusing.